
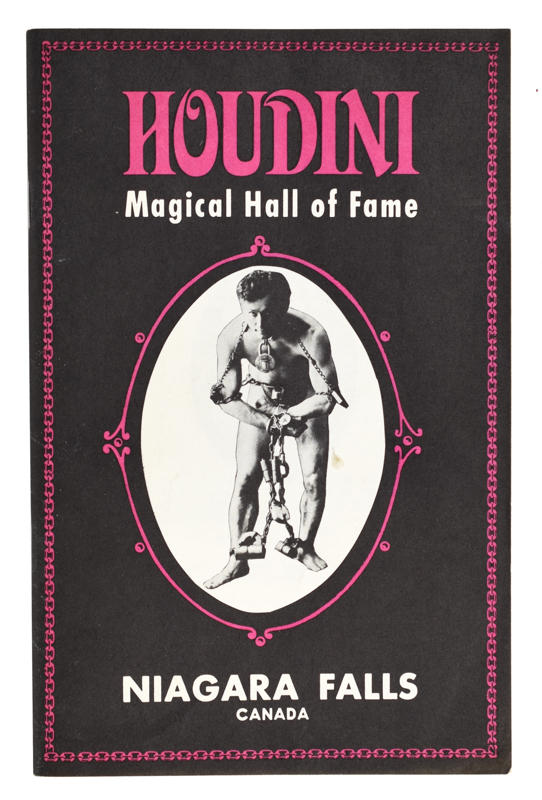
this work, we show that a large class of languages related to quadratic residuosity admits noninteractive perfect zero-knowledge proofs. The situation is no better in the interactive case where perfect zero-knowledge proofs are known only for a handful of particular languages.In. (1988), the only perfect zero-knowledge proof known was the one for quadratic nonresiduosity of Blum. In fact, since the introduction of the noninteractive model of Blum. Noninteractive perfect zero-knowledge (ZK) proofs are very elusive objects. Altogether, we argue that centrality measures do not provide solid ground for understanding the structure of psychopathology when latent confounding exists. Failing to do so risks identifying spurious relationships or failing to detect causally important effects. Our results suggest that it is essential for network psychometric approaches to examine the evidence for latent variables prior to analyzing or interpreting patterns at the symptom level. Finally, if a symptom reflects multiple latent causes, centrality metrics reflect a weighted combination, undermining their interpretability in empirical data.
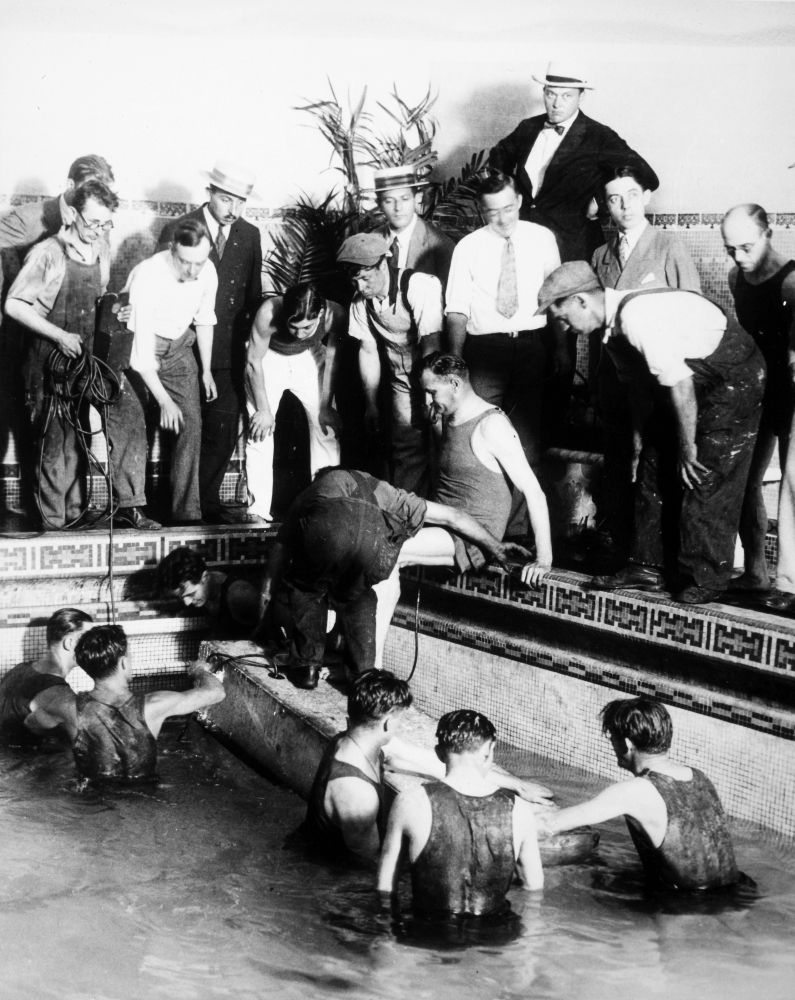
We further show that strength is redundant with factor loading in several cases. In simulations that include latent variables, we demonstrate that closeness and betweenness are vulnerable to spurious covariance among symptoms that connect subgraphs (e.g., diagnoses). The traditions of clinical taxonomy and test development in psychometric theory, however, greatly increase the possibility that latent variables exist in symptom data. In particular, common network analyses assume that there are no unmodeled latent variables that confound symptom co-occurrence. In this critical paper, we highlight conceptual and statistical problems with using centrality measures in cross-sectional networks. Do symptoms co-occur because of a latent factor, or might they directly and causally influence one another? Motivated by such questions, there has been a surge of interest in network analyses that emphasize the putatively direct role symptoms play in influencing each other. Understanding patterns of symptom co-occurrence is one of the most difficult challenges in psychopathology research.
